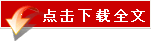
马尾松毛虫幼虫高峰期发生量 的预测模型研究
Comparison of models for forecasting peak abundance of Dendrolimus punctatus larvae
周夏芝1** 王振兴2 余 燕2 李 尚1 毕守东2*** 张国庆3 方国飞4
点击:1287次 下载:1次
DOI:10.7679/j.issn.2095-1353.2017.123
作者单位:1. 安徽农业大学林学与园林学院,合肥 230036;2. 安徽农业大学理学院,合肥 230036; 3. 安徽省潜山县林业局,潜山 246300;4. 国家林业局森林病虫害防治总站,沈阳 110034
中文关键词:马尾松毛虫幼虫高峰期发生量,平稳时间序列预测模型,回归预测模型,BP神经网络预测模型,马尔科夫链预测模型,列联表多因子多级分析预测模型
英文关键词: the peak occurrence quantity of Dendrolimus punctatus larvae, the prediction model of stationary time series, the prediction model of regression, the prediction model of BP neural network, the prediction model of Markov chains, the prediction model of contingency table analysis
中文摘要:
【目的】 为了提高马尾松毛虫Dendrolimus punctatus Walker发生量预测预报结果的准确性,为选用合适的预测模型提供依据。【方法】 本文用平稳时间序列法、回归预测法、BP神经网络法、马尔科夫链法和列联表多因子多级分析预测法研究建立安徽省潜山县1983—2016年33年的马尾松毛虫1代和2代幼虫高峰期发生量的预测模型,并对5种模型进行比较。【结果】 以卵高峰期卵量为自变量的回归模型、多元回归模型和逐步回归模型预测结果与实际值相差0.21~0.31头/株,其它8个一元回归预测结果与实际值相差1.06~1.58头/株。平稳时间序列预测2015和2016年的结果与实际值完全相符。BP神经网络预测结果若以误差标准为1头/株,1983—2014年预报准确率1代为90.32%,2代为100%。马尔科夫链预测2015和2016年,预测结果与实际值完全相符,均为1级。列联表多因子多级综合相关分析法预测2015和2016年的结果与实际值完全相符,1983—2014年1代幼虫高峰期发生量预测的历史符合率均为90.32%,2代为83.47%。为了研究不同分级标准对预测值的影响,将2代幼虫高峰期发生量的1级标准改为小于3.5头/株,则历史符合率为74.19%。【结论】 上述方法中,回归预测法自变量的选择是预报准确的关键;时间平稳序列法适用于害虫发生过程符合平稳时间序列的标准;马尔科夫链法和列联表分析法分级标准科学与否直接影响预测结果的准确性;BP神经网络法可用于自变量与预报量非线性关系的研究,是一种比较理想的预报方法。
英文摘要:
[Objectives] To improve the accuracy of forecasting the peak occurrence of Dendrolimus punctatus, and provide a foundation for choosing suitable predictive models. [Methods] Models based on five different methods; stationary time series, regression forecasting, BP neural networks, Markov chains, and contingency table analysis, were used to predict peaks of abundance of first and second generation Dendrolimus punctatus larvae over a 33 year period from 1983 to 2016 in Qianshan County Anhui Province. [Result] The single regression model used peak egg abundance as an independent variable, whereas the multiple regression and stepwise regression model used the actual 0.21 to 0.31 number of larvae per strain. The predicted and actual results of the single regression model differed by 1.06 to 1.58 larvae per strain. The actual results for 2015 and 2016 were identical to the predictions of the stationary time model for those years. If the standard error of the model based on the BP neural network was 1 larvae per strain, the accuracy of the forecast first generation from 1983 to 2014 was 90.32%, and that of the second generation was 100%. Forecasts based on Markov chains in 2015 and 2016 were highly consistent with the actual results. Forecasts based on contingency table analysis in 2015 and 2016 were all completely consistent with the actual results. The accuracy of forecasted peaks of larval abundance from 1983 to 2014 was 90.32% for the first generation, and 83.87% for the second generation. However, when the peak abundance of second generation larvae was less than 3.5 larvae per strain, then the forecast accuracy was 74.19%. [Conclusion] Choice of independent variable was the key to the accuracy of regression forecasts. Models based on stationary time series were suitable as temporal patterns of larval abundance conformed to stationary time series. Classification standards directly affect the accuracy of predictions based on contingency table analysis and Markov chains. BP neural networks can be used to investigate nonlinear relationships among independent variables and were a relatively ideal forecasting method.